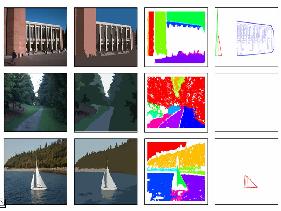
The goal of this research is to develop the necessary methodology for automated recognition of generic object and concept classes in digital images. The work builds on existing object-recognition techniques in computer vision for low-level feature extraction and designs higher-level relationship and cluster features and a new unified recognition methodology to handle the difficult problem of recognizing classes of objects, instead of particular instances. Local feature representations and global summaries that can be used by general-purpose classifiers are developed. A powerful new hierarchical multiple classifier methodology provides the learning mechanism for automating the development of recognizers for additional objects and concepts. The result of this work is a new generic object recognition paradigm that can immediately be applied to automated or semi-automated indexing of large image databases and is a step forward in object recognition.
(Faculty: Shapiro)